Wearable devices and energy expenditure
Background
As one who spent a good portion of his life being overweight, it has often been a personal struggle between my desire to lose weight and my desire to have seconds or thirds. One strategy often employed by myself (and I’m sure many others) is to use a calorie intake tracking app, such as MyFitnessPal, to achieve a negative caloric deficit and thus lose weight.
For recreational athletes hoping to manage their weight, either for performance or health, this strategy can potentially be enhanced through the addition of caloric expenditure estimates provided by wearable devices. Garmin, FitBit, Suunto, Polar, Samsung and many others produce wearable devices which give estimated caloric expenditure, either at rest or during activity. My current daily driver is a Garmin Forerunner 935. I wear it all day at work as well as for all my runs, hikes and walks. I often check the “calories” field after my runs, mostly out of curiosity as I am not currently trying to lose weight. But I started wondering, “How accurate is the caloric expenditure metric?”
What does my device tell me?
I started by taking a look at the last 313 runs I’ve done; Starting from January 1st, 2019 to the current date August 24, 2020. I then took some time to cry in the bathroom; only 313 runs in 20 months?! That number sure feels like it ought to be higher. After I dried my tears, I got to work doing a little Excel-fu. I actually did it in LibreOffice Calc, because I can’t afford Excel. But “Calc-fu” doesn’t have the same ring to it, so I just say “Excel-fu”.
Anyway, I first ran a quantitative assessment of said runs. This returned much more than I was interested in. So, I narrowed it down to mean, standard error, mode, median, standard deviation and sum of each field.
The things that stood out to me were the relatively low standard deviation and standard error for the speed and average HR fields. In other words, the majority of my runs are of similar speed and intensity. I must really need some variety in my training.
I then created a correlation matrix for each of the aforementioned data fields to see what most closely correlated to my watch’s estimated caloric expenditure.
It looks like my caloric expenditure is most closely correlated to distance and time run. Which makes sense since time is also closely correlated to distance while running. But in that case, it seems a bit odd my speed (m/s) wouldn’t be more closely correlated to calories since time and distance are both so strongly correlated to calories expended. I also thought average heart rate would be more closely correlated such that harder runs (higher heart rates) would result in greater caloric expenditure. But, wearable manufacturers are quite guarded with their predictive “special sauce” so, I have no idea what Garmin or Firstbeat take into their caloric expenditure equation. But that’s a bit outside the scope of this particular article; I guess I really don’t care how it works, just that it works as intended.
At this point, I created scatter plots of [calories vs time] and [calories vs seconds], added trend lines and did a linear regression on both.
Well, that’s pretty neat. It appears as though [Calories vs Distance] is a little more consistent as the data points are more closely clustered around the trend line. This makes sense if we’re working under the assumption more movement results in greater expenditure. Take intervals, for example; during the reps one is accumulating distance AND time, so they would both be highly correlated to calories. But, during the rest intervals, one is mostly accumulating time (assuming the runner is just slowly walking or standing in place to recover). In other words, we would want energy expenditure to go down as our accumulation of distance goes down and up as our accumulation of distance goes up.
So, it seems as though my personal watch is at least consistent with it’s estimation of my caloric expenditure. But I want to know how valid/accurate the estimation is. In order to do this, I would need some sort of “gold standard” measurement to compare my watch against. Typically, this would be something like using a gas analyzer to assess the ratio of inspired oxygen to expired carbon dioxide to calculate calories expended. While I don’t have one, many researchers do! In fact, validating energy expenditure estimations of wearable devices seems to be a fairly common topic in research.
wearables and energy expenditure
I hopped on PubMed and did a search of articles relating to validation of energy expenditure in wearable devices. I limited my search to freely available articles (hey, I’m not made out of money) published in the last five years. I also wanted to find articles assessing the widest variety of devices. I found five articles that were to my liking. There are probably many more out there, but as I mentioned previously, I limited myself to free articles and I really only have so much free time. I included a general summary of each article’s findings in Table I below but, the results were…not great.
As I would expect, some performed better than others. Some were quite a ways off; up to 43% in one study [6]. In most of the studies, the kcal estimates given by the devices where all over the place when compared to criterion measurements. Overall though, most of the devices tended to underestimate caloric expenditure. Which is a little unfortunate; I would rather have my device overestimate than underestimate.
For example, let’s say I burned 800 kcal on a run. My body knows that number, but all I have to go on is what my watch tells me. Since I’m happy where I am, I simply want to maintain my weight. Thus, I’m going to replenish the number of kcals my watch tells me I burned. If my watch says I only burned 600 kcals, I’m going to end up with a 200 kcal deficit. If my watch says I burned 1000 kcals, I’ll end up eating a 200 kcal surplus. Keep in mind, there are significant health risks to both chronic over-feeding (obesity, hypertension, diabetes) and under-feeding (stress fractures, amenorrhea, loss of muscle mass, chronic fatigue). But, given a choice, I would rather be a just a little over-fed and feeling like I can crush workouts, instead of under-fed and feeling tired and sluggish all the time. That might be one of the reasons I always felt like crap any time I would count calories when trying to lose weight. I was most likely under-eating, possibly by quite a bit.
And I know some people who read this are going to say, “Okay, so…which ones performed the best?” That was the ActiHeart 4; a chest-mounted heart rate monitor and accelerometer [3]. There are a couple caveats to this device performing best, however.
- While using “general” user anthropometrics (height, weight, sex, age, etc.) and the most recent algorithm (at the time, from 2007) the Actiheart tended to underestimate caloric expenditure during running and cycling; moreso during cycling. Caloric expenditure estimation during running was significantly improved after two changes:
- using an old algorithm (from 2005)
- after calibrating the device to the specific user with a proprietary step-test.
- This particular device is marketed as a more “clinical” assessment tool. While one can easily go to the Garmin, or Suunto, or FitBit websites and look up the price and availability of a watch, the CamNTech website simply has a “Request a quote” option. Which is kind of like going into a restaurant that doesn’t list prices on the menu; your wallet is about to be hurtin’ for certain. I couldn’t even find one of these things on eBay to get a feel for how much they could possibly cost.
In other words, I wouldn’t exactly place the Actiheart in the “consumer” category. But why did I include it then? Because it shows relatively accurate readings (without a metabolic cart) are possible. Like mentioned previously, these companies are particularly secretive about their estimation/prediction algorithms. Perhaps it would behoove them to open up a bit about what they use and how they calculate energy expenditure so others can critically assess and help enhance said algorithms. I think it will be quite some time before we end up with a prediction equation that covers all activities, at all intensities with a fairly small margin of error, but with inactivity and obesity on the rise, it’s certainly worth further investigation and development.
TABLE I: SUMMARY OF FEATURED ARTICLES
(You can click on it for a larger version)
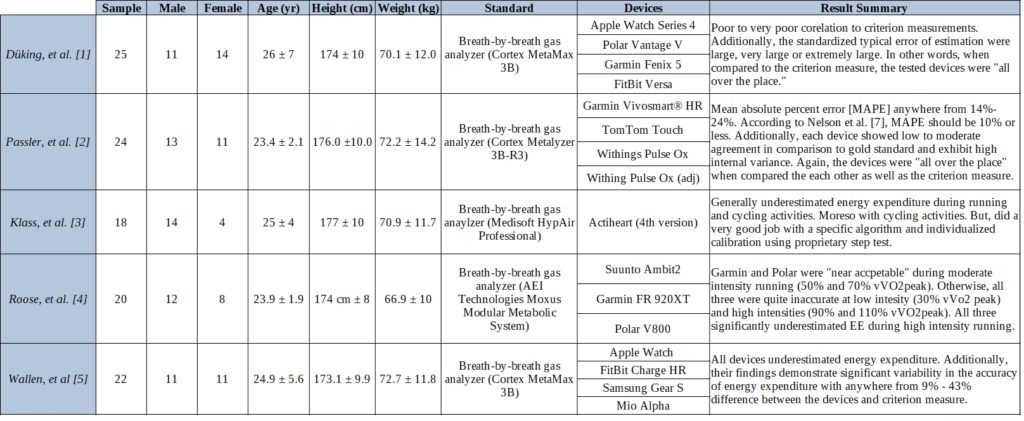
REFERENCES
- Düking P, Giessing L, Frenkel MO, Koehler K, Holmberg HC, Sperlich B. Wrist-Worn Wearables for Monitoring Heart Rate and Energy Expenditure While Sitting or Performing Light-to-Vigorous Physical Activity: Validation Study. JMIR Mhealth Uhealth. 2020;8(5):e16716. Published 2020 May 6. doi:10.2196/16716
- Passler S, Bohrer J, Blöchinger L, Senner V. Validity of Wrist-Worn Activity Trackers for Estimating VO2max and Energy Expenditure. Int J Environ Res Public Health. 2019;16(17):3037. Published 2019 Aug 22. doi:10.3390/ijerph16173037
- Klass M, Faoro V, Carpentier A. Assessment of energy expenditure during high intensity cycling and running using a heart rate and activity monitor in young active adults. PLoS One. 2019;14(11):e0224948. Published 2019 Nov 7. doi:10.1371/journal.pone.0224948
- Roos L, Taube W, Beeler N, Wyss T. Validity of sports watches when estimating energy expenditure during running. BMC Sports Sci Med Rehabil. 2017;9:22. Published 2017 Dec 20. doi:10.1186/s13102-017-0089-6
- Wallen MP, Gomersall SR, Keating SE, Wisløff U, Coombes JS. Accuracy of Heart Rate Watches: Implications for Weight Management. PLoS One. 2016;11(5):e0154420. Published 2016 May 27. doi:10.1371/journal.pone.0154420
- Nelson MB, Kaminsky LA, Dickin DC, Montoye AH. Validity of Consumer-Based Physical Activity Monitors for Specific Activity Types. Med Sci Sports Exerc. 2016;48(8):1619-1628. doi:10.1249/MSS.0000000000000933